Do you want a large or small p value?
I'll answer
Earn 20 gold coins for an accepted answer.20
Earn 20 gold coins for an accepted answer.
40more
40more
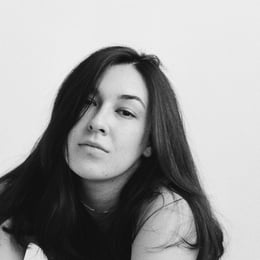
Harper Roberts
Studied at the University of Barcelona, Lives in Barcelona, Spain.
In the realm of statistical hypothesis testing, the concept of a p-value plays a crucial role in determining the validity of a statistical hypothesis. As a field expert in statistical analysis, I'd like to provide a comprehensive explanation of the significance of p-values and the implications of choosing between a large or small p-value.
Step 1: Understanding P-Values
The p-value is a statistic that measures the strength of the evidence against the null hypothesis. It is the probability of obtaining test results at least as extreme as the observed results, given that the null hypothesis is true. The null hypothesis (H0) is a statement of no effect or no difference, which is what we are testing against. The alternative hypothesis (H1 or Ha) is what we believe to be true if we reject the null hypothesis.
Significance Level (α)
When conducting a hypothesis test, researchers often set a significance level (denoted as α), which is the threshold p-value for deciding whether to reject the null hypothesis. The most common significance level is 0.05, meaning that if the p-value is less than 0.05, the evidence is considered statistically significant, and the null hypothesis is rejected. If the p-value is greater than or equal to 0.05, the evidence is not considered statistically significant, and we fail to reject the null hypothesis.
Small P-Value
A small p-value (typically ≤ 0.05) indicates that the observed data would be unlikely if the null hypothesis were true. In other words, it suggests that there is strong evidence against the null hypothesis. When the p-value is small, it implies that the results are statistically significant, and it is reasonable to conclude that the alternative hypothesis might be true. This is often interpreted as providing support for the research hypothesis.
Large P-Value
On the other hand, a large p-value (> 0.05) indicates that the observed data is not very unlikely under the assumption that the null hypothesis is true. It suggests that there is weak evidence against the null hypothesis, and the observed results could easily have occurred by chance. In this case, we do not have enough statistical evidence to reject the null hypothesis, and the results are considered statistically non-significant.
Confidence Intervals
It is also important to consider confidence intervals when interpreting p-values. A confidence interval provides a range within which we can be confident that the true population parameter lies. A narrow confidence interval indicates more precision, while a wide interval indicates less precision. If the confidence interval includes the null value (e.g., zero difference in means), it suggests that the null hypothesis cannot be rejected based on the data.
Type I and Type II Errors
When interpreting p-values, it's essential to be aware of the potential for Type I and Type II errors. A Type I error occurs when we reject the null hypothesis when it is actually true, while a Type II error occurs when we fail to reject the null hypothesis when it is false. The significance level (α) controls the probability of a Type I error, while the power of the test (1 - β) controls the probability of a Type II error.
Practical Significance
Lastly, it's important to distinguish between statistical significance and practical significance. Even if a result is statistically significant (small p-value), it may not be practically significant if the effect size is small. Conversely, a result may be practically significant but not statistically significant if the sample size is too small.
Conclusion
In summary, the choice between a large or small p-value is not a matter of preference but rather a reflection of the strength of the evidence against the null hypothesis. A small p-value provides strong evidence to reject the null hypothesis, while a large p-value indicates weak evidence, and we fail to reject the null hypothesis. It is crucial for researchers to interpret p-values in the context of their specific study, considering factors such as effect size, practical significance, and the potential for errors.
Step 1: Understanding P-Values
The p-value is a statistic that measures the strength of the evidence against the null hypothesis. It is the probability of obtaining test results at least as extreme as the observed results, given that the null hypothesis is true. The null hypothesis (H0) is a statement of no effect or no difference, which is what we are testing against. The alternative hypothesis (H1 or Ha) is what we believe to be true if we reject the null hypothesis.
Significance Level (α)
When conducting a hypothesis test, researchers often set a significance level (denoted as α), which is the threshold p-value for deciding whether to reject the null hypothesis. The most common significance level is 0.05, meaning that if the p-value is less than 0.05, the evidence is considered statistically significant, and the null hypothesis is rejected. If the p-value is greater than or equal to 0.05, the evidence is not considered statistically significant, and we fail to reject the null hypothesis.
Small P-Value
A small p-value (typically ≤ 0.05) indicates that the observed data would be unlikely if the null hypothesis were true. In other words, it suggests that there is strong evidence against the null hypothesis. When the p-value is small, it implies that the results are statistically significant, and it is reasonable to conclude that the alternative hypothesis might be true. This is often interpreted as providing support for the research hypothesis.
Large P-Value
On the other hand, a large p-value (> 0.05) indicates that the observed data is not very unlikely under the assumption that the null hypothesis is true. It suggests that there is weak evidence against the null hypothesis, and the observed results could easily have occurred by chance. In this case, we do not have enough statistical evidence to reject the null hypothesis, and the results are considered statistically non-significant.
Confidence Intervals
It is also important to consider confidence intervals when interpreting p-values. A confidence interval provides a range within which we can be confident that the true population parameter lies. A narrow confidence interval indicates more precision, while a wide interval indicates less precision. If the confidence interval includes the null value (e.g., zero difference in means), it suggests that the null hypothesis cannot be rejected based on the data.
Type I and Type II Errors
When interpreting p-values, it's essential to be aware of the potential for Type I and Type II errors. A Type I error occurs when we reject the null hypothesis when it is actually true, while a Type II error occurs when we fail to reject the null hypothesis when it is false. The significance level (α) controls the probability of a Type I error, while the power of the test (1 - β) controls the probability of a Type II error.
Practical Significance
Lastly, it's important to distinguish between statistical significance and practical significance. Even if a result is statistically significant (small p-value), it may not be practically significant if the effect size is small. Conversely, a result may be practically significant but not statistically significant if the sample size is too small.
Conclusion
In summary, the choice between a large or small p-value is not a matter of preference but rather a reflection of the strength of the evidence against the null hypothesis. A small p-value provides strong evidence to reject the null hypothesis, while a large p-value indicates weak evidence, and we fail to reject the null hypothesis. It is crucial for researchers to interpret p-values in the context of their specific study, considering factors such as effect size, practical significance, and the potential for errors.
2024-04-12 19:05:04
reply(1)
Helpful(1122)
Helpful
Helpful(2)
Works at the International Atomic Energy Agency, Lives in Vienna, Austria.
A small p-value (typically -- 0.05) indicates strong evidence against the null hypothesis, so you reject the null hypothesis. A large p-value (> 0.05) indicates weak evidence against the null hypothesis, so you fail to reject the null hypothesis.
2023-06-24 03:13:53

Carter Martin
QuesHub.com delivers expert answers and knowledge to you.
A small p-value (typically -- 0.05) indicates strong evidence against the null hypothesis, so you reject the null hypothesis. A large p-value (> 0.05) indicates weak evidence against the null hypothesis, so you fail to reject the null hypothesis.