What a P value does not tell you?
I'll answer
Earn 20 gold coins for an accepted answer.20
Earn 20 gold coins for an accepted answer.
40more
40more

Isabella Lewis
Studied at the University of Seoul, Lives in Seoul, South Korea.
As a statistical expert with a deep understanding of hypothesis testing and its implications, I often encounter misconceptions about the interpretation of p-values. Let's delve into what a p-value does not tell us, which is a crucial aspect of statistical analysis that is frequently misunderstood.
Step 1: English Answer
A p-value is a statistic that measures the strength of the evidence against the null hypothesis. It is calculated under the assumption that the null hypothesis is true and is used to determine whether or not to reject the null hypothesis in a statistical test. However, there are several things that a p-value does not tell us, and understanding these limitations is essential for proper statistical inference.
1. Magnitude of Effect: A p-value can tell you that a difference is statistically significant, but it tells you nothing about the size or magnitude of the difference. A small effect can be statistically significant if the sample size is large enough, which can lead to misleading conclusions about the practical significance of the findings.
2. Causality: The p-value does not provide any information about causal relationships. It only indicates whether there is a statistically significant association between variables. Causality requires a different set of analyses and often experimental designs that can isolate the effect of one variable on another.
3. Probability of Hypothesis Being True: It is a common misconception that a low p-value means the alternative hypothesis is true. In reality, a p-value is the probability of observing data as extreme as, or more extreme than, the test statistics under the assumption that the null hypothesis is true. It does not measure the probability that the null hypothesis is false or that the alternative hypothesis is true.
4. Error Rate: If you use an alpha level of 0.05, there's a 5% chance you will incorrectly reject the null hypothesis (Type I error). However, a p-value does not tell you the probability of making a Type II error (failing to reject a false null hypothesis), which is related to the power of the test.
5. Quality of Data: A p-value is dependent on the quality of the data collected. Poorly designed studies or those with measurement errors can lead to misleading p-values, regardless of the statistical significance.
6. Confidence in Results: A p-value does not give you a measure of confidence in the results. A result with a p-value just below the alpha threshold is not necessarily more reliable than one with a slightly higher p-value.
7.
Evidence of Truth: A p-value is not a measure of the evidence for truth. It is a measure of how incompatible the data are with the null hypothesis. A statistically significant result does not mean the alternative hypothesis is true; it simply means the data provide evidence against the null hypothesis.
8.
Replicability: A p-value does not guarantee that the results will be replicable. Replicability is a separate issue that depends on the consistency of the findings across multiple studies.
9.
Sample Representativeness: The p-value does not reflect the representativeness of the sample. A statistically significant result from a non-representative sample may not generalize to the broader population.
10.
Complexity of Real-world Phenomena: Lastly, a p-value does not account for the complexity of real-world phenomena. It is a simplified measure that assumes a certain model is correct, which may not capture all the nuances of the data.
Understanding these limitations is crucial for the proper interpretation of statistical results. It is important to view p-values as one piece of a larger puzzle, not as the sole determinant of the validity of a study's findings.
**
Step 1: English Answer
A p-value is a statistic that measures the strength of the evidence against the null hypothesis. It is calculated under the assumption that the null hypothesis is true and is used to determine whether or not to reject the null hypothesis in a statistical test. However, there are several things that a p-value does not tell us, and understanding these limitations is essential for proper statistical inference.
1. Magnitude of Effect: A p-value can tell you that a difference is statistically significant, but it tells you nothing about the size or magnitude of the difference. A small effect can be statistically significant if the sample size is large enough, which can lead to misleading conclusions about the practical significance of the findings.
2. Causality: The p-value does not provide any information about causal relationships. It only indicates whether there is a statistically significant association between variables. Causality requires a different set of analyses and often experimental designs that can isolate the effect of one variable on another.
3. Probability of Hypothesis Being True: It is a common misconception that a low p-value means the alternative hypothesis is true. In reality, a p-value is the probability of observing data as extreme as, or more extreme than, the test statistics under the assumption that the null hypothesis is true. It does not measure the probability that the null hypothesis is false or that the alternative hypothesis is true.
4. Error Rate: If you use an alpha level of 0.05, there's a 5% chance you will incorrectly reject the null hypothesis (Type I error). However, a p-value does not tell you the probability of making a Type II error (failing to reject a false null hypothesis), which is related to the power of the test.
5. Quality of Data: A p-value is dependent on the quality of the data collected. Poorly designed studies or those with measurement errors can lead to misleading p-values, regardless of the statistical significance.
6. Confidence in Results: A p-value does not give you a measure of confidence in the results. A result with a p-value just below the alpha threshold is not necessarily more reliable than one with a slightly higher p-value.
7.
Evidence of Truth: A p-value is not a measure of the evidence for truth. It is a measure of how incompatible the data are with the null hypothesis. A statistically significant result does not mean the alternative hypothesis is true; it simply means the data provide evidence against the null hypothesis.
8.
Replicability: A p-value does not guarantee that the results will be replicable. Replicability is a separate issue that depends on the consistency of the findings across multiple studies.
9.
Sample Representativeness: The p-value does not reflect the representativeness of the sample. A statistically significant result from a non-representative sample may not generalize to the broader population.
10.
Complexity of Real-world Phenomena: Lastly, a p-value does not account for the complexity of real-world phenomena. It is a simplified measure that assumes a certain model is correct, which may not capture all the nuances of the data.
Understanding these limitations is crucial for the proper interpretation of statistical results. It is important to view p-values as one piece of a larger puzzle, not as the sole determinant of the validity of a study's findings.
**
2024-04-15 09:18:44
reply(1)
Helpful(1122)
Helpful
Helpful(2)
Works at the International Committee of the Red Cross, Lives in Geneva, Switzerland.
A p-value can tell you that a difference is statistically significant, but it tells you nothing about the size or magnitude of the difference. "The p-value is low, so the alternative hypothesis is true." ... If you use an alpha level of 0.05, there's a 5% chance you will incorrectly reject the null hypothesis.Jun 20, 2011
2023-06-21 03:13:52
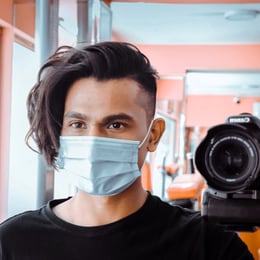
Lucas Harris
QuesHub.com delivers expert answers and knowledge to you.
A p-value can tell you that a difference is statistically significant, but it tells you nothing about the size or magnitude of the difference. "The p-value is low, so the alternative hypothesis is true." ... If you use an alpha level of 0.05, there's a 5% chance you will incorrectly reject the null hypothesis.Jun 20, 2011