How do you interpret a 95 confidence interval?
I'll answer
Earn 20 gold coins for an accepted answer.20
Earn 20 gold coins for an accepted answer.
40more
40more
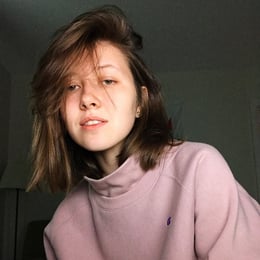
Lily Gonzales
Studied at the University of Toronto, Lives in Toronto, Canada.
As a statistical expert with extensive experience in data analysis and interpretation, I often encounter the concept of confidence intervals in my work. A confidence interval is a range of values, derived from a data set, that is used to estimate an unknown population parameter. When we talk about a 95% confidence interval, we are referring to a specific level of confidence that the true population parameter lies within this range. Here's a detailed interpretation:
### Understanding the 95% Confidence Interval
1. Statistical Inference: The confidence interval is a fundamental tool in statistical inference. It allows us to make inferences about a population based on a sample.
2. Estimation: It provides an estimate of the population parameter, such as the mean, with an associated level of uncertainty.
3. Probability: The probability associated with the confidence interval (in this case, 95%) is not a probability that the parameter is in the interval, but rather a probability of the method used to construct the interval. It's a long-run frequency interpretation; if we were to take many samples and construct a confidence interval from each, we expect that 95% of those intervals would contain the true population parameter.
4. Not a Range of Possibility: It's important to note that a 95% confidence interval does not mean that there is a 95% chance that the parameter is within the interval. This is a common misconception. The parameter is either in the interval or it is not; it's not a matter of probability for the parameter itself.
5. Sampling Distribution: The confidence interval is based on the sampling distribution of the statistic used to estimate the parameter. For the mean, this would be the distribution of the sample means if we were to take many samples.
6. Margin of Error: The interval includes a margin of error, which accounts for the uncertainty of the estimate. The margin of error decreases as the sample size increases, assuming the standard deviation of the population is known.
7.
Parameters vs. Statistics: A key distinction is that confidence intervals are about population parameters, not sample statistics. The sample mean is a statistic; the population mean is a parameter.
8.
Confidence Level: The level of confidence (95% in this case) is chosen based on how much uncertainty we are willing to tolerate. A higher confidence level (e.g., 99%) would provide a wider interval that is less precise but more certain.
9.
Coverage Error: There is a 5% chance, known as the coverage error, that the true population parameter does not fall within the calculated confidence interval. This is not a flaw but an inherent part of the statistical process.
10.
Not a Prediction Interval: A confidence interval is not the same as a prediction interval. A prediction interval is used to predict future observations, while a confidence interval is used to estimate a population parameter.
1
1. Symmetric vs. Asymmetric: Confidence intervals are often symmetric around the sample statistic, but they can also be asymmetric, especially in the case of non-normal distributions or when using certain statistical methods.
1
2. Context Matters: The interpretation of a confidence interval must always be made in the context of the research question and the design of the study.
### Conclusion
In summary, a 95% confidence interval is a statistical tool that provides an estimated range for an unknown population parameter with a certain level of confidence. It is crucial to understand the nuances of what this interval represents and the assumptions behind its calculation to correctly interpret and communicate the results of statistical analyses.
### Understanding the 95% Confidence Interval
1. Statistical Inference: The confidence interval is a fundamental tool in statistical inference. It allows us to make inferences about a population based on a sample.
2. Estimation: It provides an estimate of the population parameter, such as the mean, with an associated level of uncertainty.
3. Probability: The probability associated with the confidence interval (in this case, 95%) is not a probability that the parameter is in the interval, but rather a probability of the method used to construct the interval. It's a long-run frequency interpretation; if we were to take many samples and construct a confidence interval from each, we expect that 95% of those intervals would contain the true population parameter.
4. Not a Range of Possibility: It's important to note that a 95% confidence interval does not mean that there is a 95% chance that the parameter is within the interval. This is a common misconception. The parameter is either in the interval or it is not; it's not a matter of probability for the parameter itself.
5. Sampling Distribution: The confidence interval is based on the sampling distribution of the statistic used to estimate the parameter. For the mean, this would be the distribution of the sample means if we were to take many samples.
6. Margin of Error: The interval includes a margin of error, which accounts for the uncertainty of the estimate. The margin of error decreases as the sample size increases, assuming the standard deviation of the population is known.
7.
Parameters vs. Statistics: A key distinction is that confidence intervals are about population parameters, not sample statistics. The sample mean is a statistic; the population mean is a parameter.
8.
Confidence Level: The level of confidence (95% in this case) is chosen based on how much uncertainty we are willing to tolerate. A higher confidence level (e.g., 99%) would provide a wider interval that is less precise but more certain.
9.
Coverage Error: There is a 5% chance, known as the coverage error, that the true population parameter does not fall within the calculated confidence interval. This is not a flaw but an inherent part of the statistical process.
10.
Not a Prediction Interval: A confidence interval is not the same as a prediction interval. A prediction interval is used to predict future observations, while a confidence interval is used to estimate a population parameter.
1
1. Symmetric vs. Asymmetric: Confidence intervals are often symmetric around the sample statistic, but they can also be asymmetric, especially in the case of non-normal distributions or when using certain statistical methods.
1
2. Context Matters: The interpretation of a confidence interval must always be made in the context of the research question and the design of the study.
### Conclusion
In summary, a 95% confidence interval is a statistical tool that provides an estimated range for an unknown population parameter with a certain level of confidence. It is crucial to understand the nuances of what this interval represents and the assumptions behind its calculation to correctly interpret and communicate the results of statistical analyses.
2024-05-08 22:26:14
reply(1)
Helpful(1122)
Helpful
Helpful(2)
Works at the Red Cross, Lives in Geneva, Switzerland.
If repeated samples were taken and the 95% confidence interval was computed for each sample, 95% of the intervals would contain the population mean. A 95% confidence interval has a 0.95 probability of containing the population mean. 95% of the population distribution is contained in the confidence interval.
2023-06-16 20:51:36
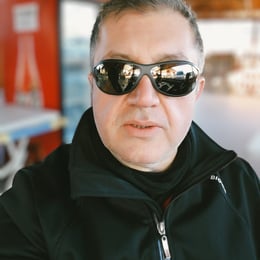
Oliver Cooper
QuesHub.com delivers expert answers and knowledge to you.
If repeated samples were taken and the 95% confidence interval was computed for each sample, 95% of the intervals would contain the population mean. A 95% confidence interval has a 0.95 probability of containing the population mean. 95% of the population distribution is contained in the confidence interval.