What is the 95% confidence level?
I'll answer
Earn 20 gold coins for an accepted answer.20
Earn 20 gold coins for an accepted answer.
40more
40more
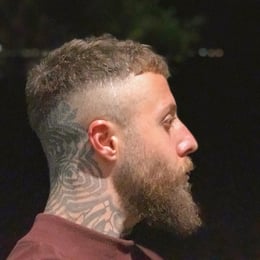
Julian Patel
Works at the International Development Association, Lives in Washington, D.C., USA.
As an expert in statistical analysis, I can explain the concept of a 95% confidence level in detail. In statistics, a confidence level is a term used to describe the level of confidence that we have that a given statistical interval, known as a confidence interval, contains the true value of a population parameter. The population parameter is a characteristic of the entire population that we are interested in estimating, such as the mean (average), proportion, or variance.
When we say that we are working with a 95% confidence level, we are essentially stating that if we were to take multiple samples from the population and calculate a confidence interval for each sample, we would expect that 95% of those intervals would contain the true population parameter. It is important to note that this does not mean that there is a 95% chance that the parameter is within the interval for a single sample; rather, it is the proportion of intervals that would contain the parameter if the process were repeated many times.
The calculation of a confidence interval typically involves two components: the sample statistic (such as the sample mean) and the margin of error. The margin of error is determined by the level of confidence we want to achieve and the variability within the data. The higher the confidence level, the larger the margin of error must be to account for the increased certainty.
The formula for calculating a confidence interval for a mean is generally as follows:
\[ CI = \bar{x} \pm (Z \times \frac{s}{\sqrt{n}}) \]
Where:
- \( CI \) is the confidence interval.
- \( \bar{x} \) is the sample mean.
- \( Z \) is the z-score corresponding to the desired confidence level (for a 95% confidence level, the z-score is approximately 1.96).
- \( s \) is the sample standard deviation.
- \( n \) is the sample size.
The z-score is a measure of how many standard deviations an observation or data point is from the mean. For a 95% confidence level, the z-score is derived from the standard normal distribution, which is a bell-shaped curve that represents the distribution of sample means when the population is normally distributed.
It is crucial to understand that a confidence interval provides a range of values within which we can be confident the true population parameter lies. It is not a range of possible values for the parameter itself, but rather a range of plausible values for the parameter based on the data we have.
In practice, confidence intervals are used in various fields, including social sciences, biological sciences, and engineering, to make inferences about populations based on sample data. They are a fundamental tool in hypothesis testing and in the analysis of data from surveys and experiments.
It is also worth mentioning that the choice of a confidence level (such as 95%) is somewhat arbitrary and depends on the context of the study and the acceptable level of risk for being incorrect. A higher confidence level (such as 99%) would provide a more precise estimate but would require a larger sample size or a wider interval to compensate for the increased certainty.
In summary, a 95% confidence level is a statistical concept that indicates the probability of capturing the true population parameter within the calculated confidence interval when the process of sampling and interval estimation is repeated a large number of times. It is a measure of the reliability of our estimate and is a key component in statistical inference.
When we say that we are working with a 95% confidence level, we are essentially stating that if we were to take multiple samples from the population and calculate a confidence interval for each sample, we would expect that 95% of those intervals would contain the true population parameter. It is important to note that this does not mean that there is a 95% chance that the parameter is within the interval for a single sample; rather, it is the proportion of intervals that would contain the parameter if the process were repeated many times.
The calculation of a confidence interval typically involves two components: the sample statistic (such as the sample mean) and the margin of error. The margin of error is determined by the level of confidence we want to achieve and the variability within the data. The higher the confidence level, the larger the margin of error must be to account for the increased certainty.
The formula for calculating a confidence interval for a mean is generally as follows:
\[ CI = \bar{x} \pm (Z \times \frac{s}{\sqrt{n}}) \]
Where:
- \( CI \) is the confidence interval.
- \( \bar{x} \) is the sample mean.
- \( Z \) is the z-score corresponding to the desired confidence level (for a 95% confidence level, the z-score is approximately 1.96).
- \( s \) is the sample standard deviation.
- \( n \) is the sample size.
The z-score is a measure of how many standard deviations an observation or data point is from the mean. For a 95% confidence level, the z-score is derived from the standard normal distribution, which is a bell-shaped curve that represents the distribution of sample means when the population is normally distributed.
It is crucial to understand that a confidence interval provides a range of values within which we can be confident the true population parameter lies. It is not a range of possible values for the parameter itself, but rather a range of plausible values for the parameter based on the data we have.
In practice, confidence intervals are used in various fields, including social sciences, biological sciences, and engineering, to make inferences about populations based on sample data. They are a fundamental tool in hypothesis testing and in the analysis of data from surveys and experiments.
It is also worth mentioning that the choice of a confidence level (such as 95%) is somewhat arbitrary and depends on the context of the study and the acceptable level of risk for being incorrect. A higher confidence level (such as 99%) would provide a more precise estimate but would require a larger sample size or a wider interval to compensate for the increased certainty.
In summary, a 95% confidence level is a statistical concept that indicates the probability of capturing the true population parameter within the calculated confidence interval when the process of sampling and interval estimation is repeated a large number of times. It is a measure of the reliability of our estimate and is a key component in statistical inference.
2024-05-08 22:26:51
reply(1)
Helpful(1122)
Helpful
Helpful(2)
Studied at Stanford University, Lives in Palo Alto, CA
If repeated samples were taken and the 95% confidence interval was computed for each sample, 95% of the intervals would contain the population mean. A 95% confidence interval has a 0.95 probability of containing the population mean.
2023-06-10 20:51:30
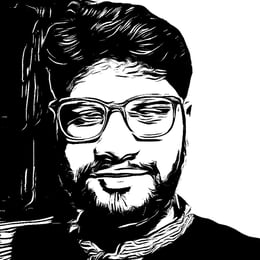
Lucas Carter
QuesHub.com delivers expert answers and knowledge to you.
If repeated samples were taken and the 95% confidence interval was computed for each sample, 95% of the intervals would contain the population mean. A 95% confidence interval has a 0.95 probability of containing the population mean.