Is T one to one?
I'll answer
Earn 20 gold coins for an accepted answer.20
Earn 20 gold coins for an accepted answer.
40more
40more
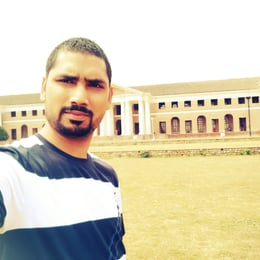
William Adams
Works at Google, Lives in Mountain View, CA
As a domain expert in the field of linear algebra, I can provide a detailed explanation regarding the concept of one-to-one transformations, also known as injective maps, and how they relate to the properties of matrices and transformations.
To begin with, let's define what a one-to-one transformation is. A transformation \( T: V \rightarrow W \) from a vector space \( V \) to a vector space \( W \) is said to be one-to-one (or injective) if for every pair of distinct vectors \( \mathbf{u}, \mathbf{v} \in V \), their images under \( T \) are also distinct, i.e., \( T(\mathbf{u}) \neq T(\mathbf{v}) \). This means that no two different vectors in \( V \) can map to the same vector in \( W \) under \( T \).
Now, let's consider a linear transformation \( T: \mathbb{R}^n \rightarrow \mathbb{R}^m \) represented by a matrix \( A \) of size \( m \times n \). The transformation \( T \) can be expressed as \( T(\mathbf{x}) = A\mathbf{x} \), where \( \mathbf{x} \) is a vector in \( \mathbb{R}^n \).
The statement "Thus u = v. So if T maps two vectors to the same output, those two vectors are really the same vector. Thus, T is one-to-one." suggests a misunderstanding. The correct interpretation is that if \( T(\mathbf{u}) = T(\mathbf{v}) \), then \( \mathbf{u} \) and \( \mathbf{v} \) must be the same vector for \( T \) to be one-to-one. However, the converse is not necessarily true; just because two vectors are the same does not imply that the transformation is one-to-one.
To determine if \( T \) is one-to-one, we can look at the null space of \( T \). The null space is the set of all vectors \( \mathbf{x} \) such that \( T(\mathbf{x}) = \mathbf{0} \), where \( \mathbf{0} \) is the zero vector in \( \mathbb{R}^m \). If the null space of \( T \) contains only the zero vector, then \( T \) is one-to-one. This is because if \( T(\mathbf{u}) = T(\mathbf{v}) \), then \( A\mathbf{u} = A\mathbf{v} \), which implies \( A(\mathbf{u} - \mathbf{v}) = \mathbf{0} \). If the only solution to this equation is \( \mathbf{u} - \mathbf{v} = \mathbf{0} \), or equivalently \( \mathbf{u} = \mathbf{v} \), then \( T \) is indeed one-to-one.
The condition provided in the reference material, "(b) T is a one-to-one transformation if and only if the columns of A are linearly independent," is indeed correct. Linear independence of the columns of \( A \) is a necessary and sufficient condition for \( T \) to be one-to-one. If the columns of \( A \) are linearly independent, then no non-trivial linear combination of the columns can result in the zero vector, which means that the only vector that maps to the zero vector under \( T \) is the zero vector itself.
On the other hand, the statement "(a) T is an onto transformation if and only if the columns of A span \( \mathbb{R}^m \)" is also correct but pertains to a different property of transformations, known as onto or surjective. An onto transformation means that for every vector \( \mathbf{y} \) in \( \mathbb{R}^m \), there exists at least one vector \( \mathbf{x} \) in \( \mathbb{R}^n \) such that \( T(\mathbf{x}) = \mathbf{y} \). This is equivalent to saying that the columns of \( A \) must span the entire space \( \mathbb{R}^m \), which implies that \( m \leq n \) and the columns of \( A \) form a basis for \( \mathbb{R}^m \).
In summary, a linear transformation \( T \) represented by a matrix \( A \) is one-to-one if and only if the columns of \( A \) are linearly independent, which ensures that the null space of \( T \) contains only the zero vector. This property is distinct from being onto, which requires the columns of \( A \) to span \( \mathbb{R}^m \) and is only possible if the number of columns in \( A \) is at least as large as the dimension of \( \mathbb{R}^m \).
To begin with, let's define what a one-to-one transformation is. A transformation \( T: V \rightarrow W \) from a vector space \( V \) to a vector space \( W \) is said to be one-to-one (or injective) if for every pair of distinct vectors \( \mathbf{u}, \mathbf{v} \in V \), their images under \( T \) are also distinct, i.e., \( T(\mathbf{u}) \neq T(\mathbf{v}) \). This means that no two different vectors in \( V \) can map to the same vector in \( W \) under \( T \).
Now, let's consider a linear transformation \( T: \mathbb{R}^n \rightarrow \mathbb{R}^m \) represented by a matrix \( A \) of size \( m \times n \). The transformation \( T \) can be expressed as \( T(\mathbf{x}) = A\mathbf{x} \), where \( \mathbf{x} \) is a vector in \( \mathbb{R}^n \).
The statement "Thus u = v. So if T maps two vectors to the same output, those two vectors are really the same vector. Thus, T is one-to-one." suggests a misunderstanding. The correct interpretation is that if \( T(\mathbf{u}) = T(\mathbf{v}) \), then \( \mathbf{u} \) and \( \mathbf{v} \) must be the same vector for \( T \) to be one-to-one. However, the converse is not necessarily true; just because two vectors are the same does not imply that the transformation is one-to-one.
To determine if \( T \) is one-to-one, we can look at the null space of \( T \). The null space is the set of all vectors \( \mathbf{x} \) such that \( T(\mathbf{x}) = \mathbf{0} \), where \( \mathbf{0} \) is the zero vector in \( \mathbb{R}^m \). If the null space of \( T \) contains only the zero vector, then \( T \) is one-to-one. This is because if \( T(\mathbf{u}) = T(\mathbf{v}) \), then \( A\mathbf{u} = A\mathbf{v} \), which implies \( A(\mathbf{u} - \mathbf{v}) = \mathbf{0} \). If the only solution to this equation is \( \mathbf{u} - \mathbf{v} = \mathbf{0} \), or equivalently \( \mathbf{u} = \mathbf{v} \), then \( T \) is indeed one-to-one.
The condition provided in the reference material, "(b) T is a one-to-one transformation if and only if the columns of A are linearly independent," is indeed correct. Linear independence of the columns of \( A \) is a necessary and sufficient condition for \( T \) to be one-to-one. If the columns of \( A \) are linearly independent, then no non-trivial linear combination of the columns can result in the zero vector, which means that the only vector that maps to the zero vector under \( T \) is the zero vector itself.
On the other hand, the statement "(a) T is an onto transformation if and only if the columns of A span \( \mathbb{R}^m \)" is also correct but pertains to a different property of transformations, known as onto or surjective. An onto transformation means that for every vector \( \mathbf{y} \) in \( \mathbb{R}^m \), there exists at least one vector \( \mathbf{x} \) in \( \mathbb{R}^n \) such that \( T(\mathbf{x}) = \mathbf{y} \). This is equivalent to saying that the columns of \( A \) must span the entire space \( \mathbb{R}^m \), which implies that \( m \leq n \) and the columns of \( A \) form a basis for \( \mathbb{R}^m \).
In summary, a linear transformation \( T \) represented by a matrix \( A \) is one-to-one if and only if the columns of \( A \) are linearly independent, which ensures that the null space of \( T \) contains only the zero vector. This property is distinct from being onto, which requires the columns of \( A \) to span \( \mathbb{R}^m \) and is only possible if the number of columns in \( A \) is at least as large as the dimension of \( \mathbb{R}^m \).
2024-05-13 15:10:04
reply(1)
Helpful(1122)
Helpful
Helpful(2)
Works at the International Monetary Fund, Lives in Washington, D.C., USA.
Thus u = v. So if T maps two vectors to the same output, those two vectors are really the same vector. Thus, T is one-to-one. (a) T is an onto transformation if and only if the columns of A span Rm and (b) T is a one-to-one transformation if and only if the columns of A are linearly independent.
2023-06-12 22:31:59

Mia Wright
QuesHub.com delivers expert answers and knowledge to you.
Thus u = v. So if T maps two vectors to the same output, those two vectors are really the same vector. Thus, T is one-to-one. (a) T is an onto transformation if and only if the columns of A span Rm and (b) T is a one-to-one transformation if and only if the columns of A are linearly independent.